What is Artificial Intelligence?
Artificial intelligence (AI) can be defined in various ways – each perspective carrying implications for how we understand, develop, and use these technologies in education. Take for instance the definitions of the two words in the term:
artificial (ar·ti·fi·cial) [adj]:
made, produced, or done by humans especially to seem like something natural : man-made
intelligence (in·tel·li·gence) [noun]:
- the ability to learn or understand or to deal with new or trying situations : REASON
also : the skilled use of reason - the ability to apply knowledge to manipulate one’s environment or to think abstractly as measured by objective criteria (such as tests)
Table of Contents
These two definitions underscore different emphases: a focus on the human-defined nature of AI and a focus on the human-like nature of AI. If we explore commonly used definitions of AI, we can explore these different perspectives more.
Depending on which definition (or combination thereof) you adopt, you may focus more on AI’s resemblance to human cognition or on human-driven design and objectives. Recognizing that these dimensions coexist is crucial for understanding AI’s growing role in our schools and communities – and how we can monitor and regulate the impact it may have.
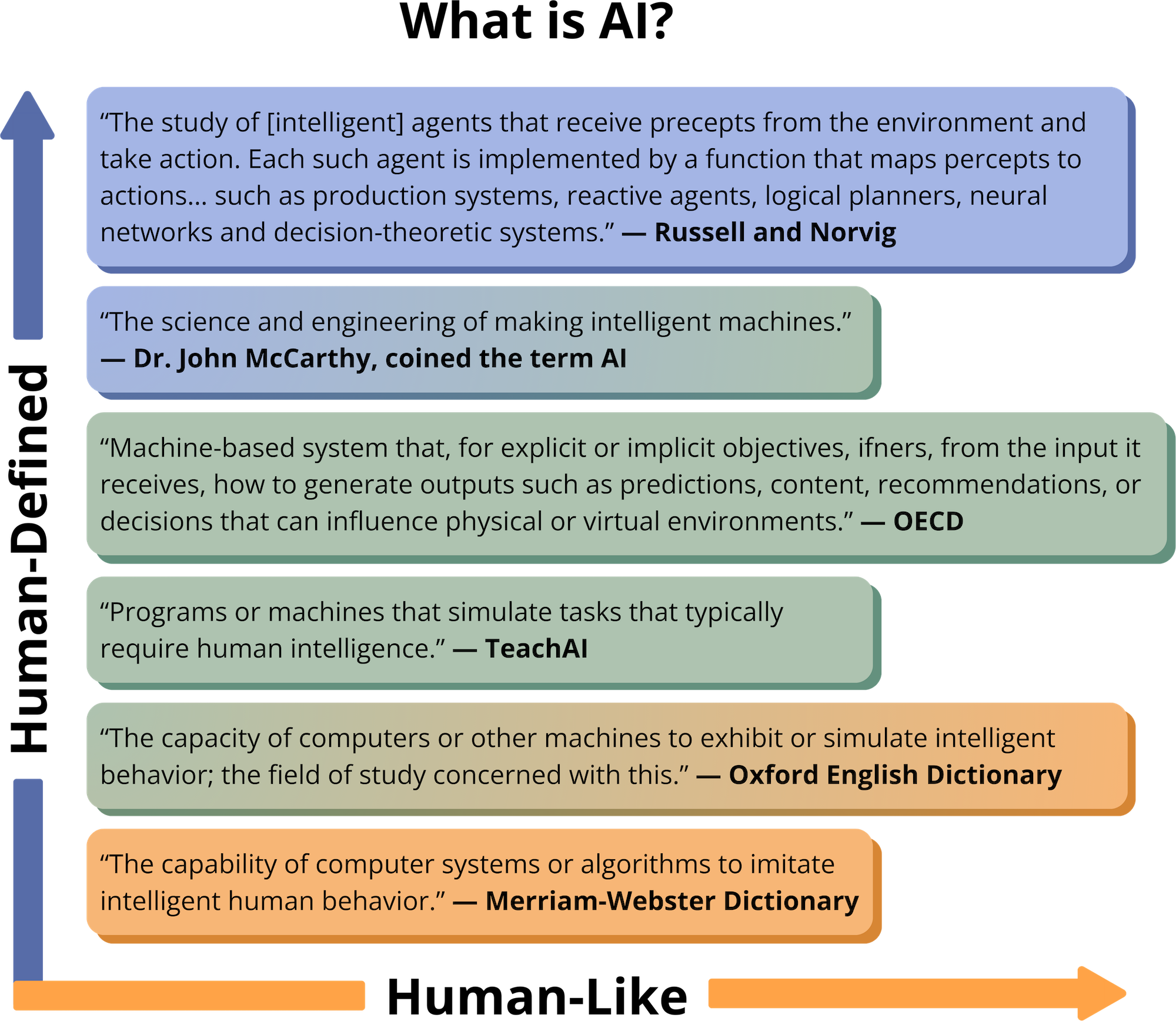
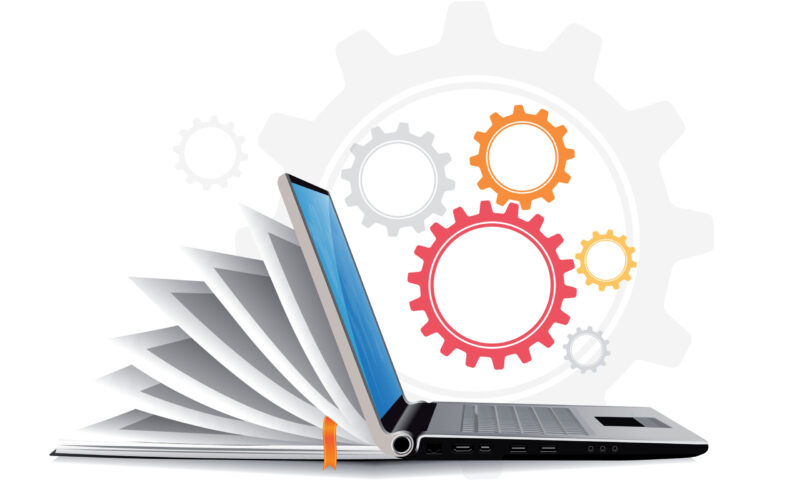
Florida K-12 AI Task Force Definition of AI
Taking both the “human-like” and “human-defined” approaches into account, we propose a working definition for this document:
“AI refers to human-created, machine-based systems that simulate tasks typically requiring human intelligence, including decision-making, predictions, content generation, and recommendations.”
This definition stresses that human creativity and oversight guide AI, even as these systems tackle increasingly sophisticated tasks once performed exclusively by people.
A Brief Timeline of AI in Education
How is AI used in education?
At the time of writing this, there are over 500 apps that utilize AI with the goal of supporting education – and the number continues to grow. This list is not intended to demonstrate longitudinal success of each application and all approaches carry inherent risks that require careful monitoring and evaluation. For now, we are providing a few example approaches!
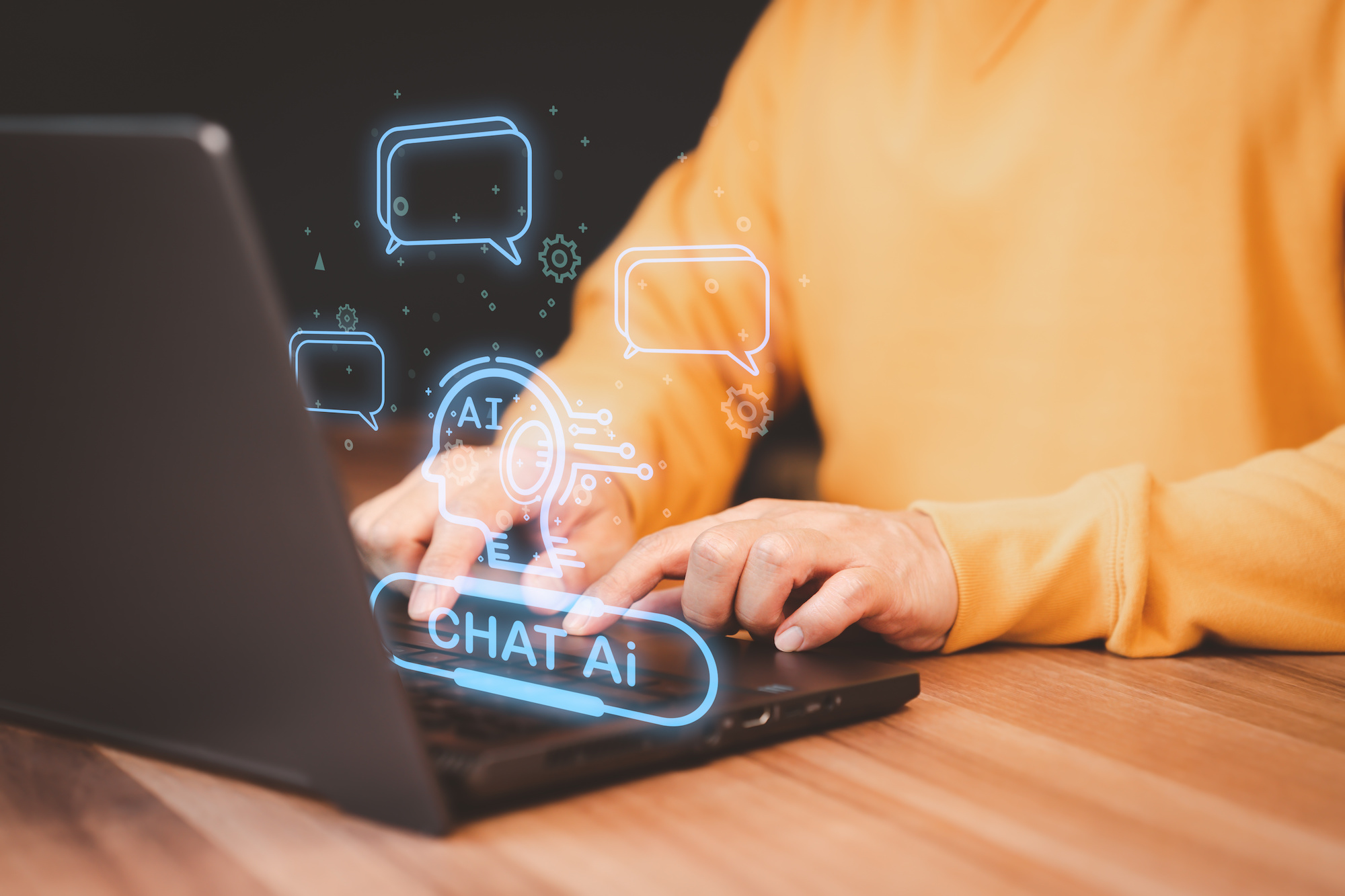
Classroom Instruction
- Dictation (Speech-to-Text): Assists students with writing challenges or needing accommodations.
- Grammar & Spelling Suggestions: Provides real-time feedback on writing, guiding students to refine their work.
- Plagiarism Checkers: Flags potential unoriginal content* and promotes academic honesty.
- Live Captions & Translations: Generates captions for presentations, videos, or audio recordings, enhancing accessibility.
- Background Noise Cancellation: Improves audio quality during virtual sessions or recorded lessons.
- Adaptive Texts & Assessments: Dynamically adjusts difficulty or reading levels based on individual performance.
- Predictive Text & Text Generation: Offers word or phrase suggestions, and, in some cases, full text-generation for writing support (e.g., essay drafts, summaries).
- Text-to-Speech: Converts written text into audio, aiding students with reading disabilities or visual impairments.
- Design Suggestions: Proposes slide layouts and graphics in tools like Google Slides or Microsoft PowerPoint.
- Real-Time Fluency Trackers: Monitors pronunciation, pacing, and intonation in language acquisition or public speaking.
- Translation Tools for Text, Speech, & Images: Breaks language barriers in multilingual classrooms.
- Personalized Tutoring & Automated Feedback: Leverages AI to deliver individualized instruction, practice exercises, and instant feedback on assignments, supporting deeper mastery of content.
- Cross-application integration: Streamlines productivity by enabling communication between AI tools and other applications, such as syncing AI-generated content directly into design tools, spreadsheets, collaborative documents, etc.
Formative feedback: Leveraging AI and chatbots in lessons or group activities to provide instant formative feedback, answer common questions, and guide students through challenges, allowing teachers to focus on providing in-depth support to students or groups requiring additional assistance.
School Safety & Security
- Real-Time Threat Detection: Uses computer vision to analyze live camera feeds for suspicious activities.
- Visitor Management Systems: Automates identity checks, ensuring only authorized personnel enter school grounds.
Emergency Alert Protocols: Integrates AI-driven notifications to facilitate swift crisis response and evacuation procedures.
Educator Support
- AI Coaching Tools: Analyzes classroom video or audio to offer feedback on instructional methods.
- Virtual PD Sessions: Utilizes adaptive learning platforms to personalize professional development for teachers.
- Collaboration Platforms: Suggests research articles, or resources based on needs and goals.
- AI-Driven Lesson Planning: Generates lesson outlines, activity ideas, and differentiation strategies based on curriculum standards.
- Leveraging Efficiency to Augment Instruction: Automating tasks like grading and attendance tracking, allowing educators to focus more on instruction and student engagement.
Productivity Assistant: Generates emails, newsletters, rubrics, images, and more, reducing the operational load on teachers to allow more time for instructional activities, classroom management, and work-life balance.
Data Analytics & Policy Planning
- Learning Analytics Dashboards: Aggregates and visualizes student performance data for instructional insights.
- Longitudinal Tracking: Monitors student progress over time, informing curriculum updates and targeted interventions.
- Student Attendance: Identifying trends, predicting absenteeism risks, and providing actionable insights for early interventions, helping schools develop targeted policies to improve student engagement and reduce chronic absenteeism.
Policy Development: Leverages large datasets to inform district or state education strategies, with a focus on equity and evidence-based practices.
Facilities & School Building Management
- Smart Heating, Ventilation, & Air Conditioning (HVAC): Adjusts energy usage based on occupancy patterns, saving costs and improving comfort.
- Lighting & Energy Optimization: Employs sensors and AI algorithms to reduce power consumption effectively.
IoT Device Coordination: Monitors and troubleshoots networked devices (e.g., interactive boards).
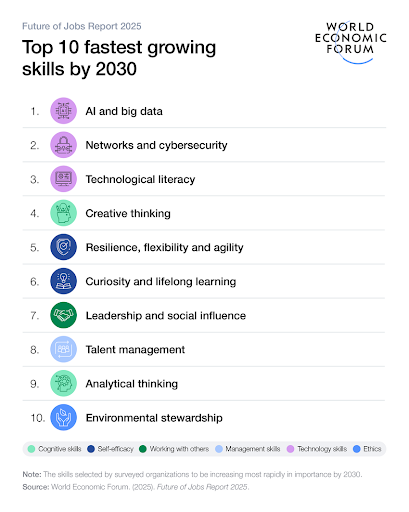
Preparing Florida for the Future of Work
The World Economic Forum’s Future of Jobs 2025 report underscores the significance of AI-related skills for thriving in the workforce by 2030 – adding additional motivation for systematically, effectively, and safely integrating AI literacy and tools into our schools. While it is crucial to prepare students—particularly high school graduates—with these competencies, it is equally important to establish robust professional development pathways for educators and staff. Equipping them with the necessary upskilling opportunities empowers current teachers and community leaders to drive meaningful change and foster a future-ready learning environment.
One approach to meet these needs is to establish a School Community Skills-Focused Checklist, created with teachers, staff, students, and parents/guardians, to identify ways in which community members are being offered opportunities to learn and implement these skills. For instance, for Curiosity and Lifelong Learning: In what ways do curricula at your school emphasize self-directed learning, reflection, and the ability to adapt skill sets using AI-enhanced technologies? Are there clear pathways for ongoing upskilling of your teachers and staff in emerging AI and digital tools?
Key AI in Education Terminology
A
- Adaptive Learning
Using artificial intelligence to personalize educational experiences by tailoring content and pacing to each student’s needs based on student’s performance on tasks, learning behaviors, response times, and interactions within the learning platform. - AI Literacy
The knowledge and skills required to understand and interact with artificial intelligence technologies effectively and responsibly. - Algorithm
A set of step-by-step instructions or rules used by computers to solve problems or perform tasks. - Anonymized Data
Data that has been stripped of all personally identifiable information (PII), making it impossible to trace back to an individual. - Artificial Intelligence
Human-created, machine-based systems that simulate tasks that typically require human intelligence, including decision-making, predictions, content generation, and recommendations. - Artificial General Intelligence (AGI)
A type of artificial intelligence that has the ability to understand, learn, and apply knowledge across a wide range of tasks, similar to human intelligence. In theory, AGI can perform any intellectual task that a human being can do, demonstrating flexibility, creativity, and adaptability in problem-solving and decision-making. However, we aren’t there yet! - Artificial Narrow Intelligence (ANI)
A type of artificial intelligence designed to perform a specific task or a narrow range of tasks very well. Unlike human intelligence, which is versatile and can handle a wide variety of activities, ANI is limited to its programmed functions, such as voice recognition, image classification, or playing a game like chess. - Augmented Reality (AR)
The integration of artificial intelligence with real-world environments to overlay digital content, often used in educational applications. - Automation
Using artificial intelligence to perform repetitive or mundane tasks, freeing up time for more complex or creative activities.
B
- Bias
Unfair or inaccurate outcomes in artificial intelligence systems caused by skewed or incomplete training data, leading to discriminatory or unbalanced results. - Big Data
Extremely large datasets that require specialized tools and methods for analysis. Artificial intelligence can use big data to uncover patterns and insights.
C
- Chain-of-Thought Prompting
Encouraging artificial intelligence to break down complex tasks step by step. - Chatbot
An artificial intelligence application that simulates conversation with users, often used for customer service or educational purposes. - Classification
An artificial intelligence task where data is categorized into predefined groups, such as identifying spam emails or sorting student submissions. - Clustering
An artificial intelligence technique used to group similar data points together without predefined categories, often part of unsupervised learning. - Computer Vision
An area of artificial intelligence that enables machines to interpret and make decisions based on visual inputs, such as images or videos.
D
- Data
Information collected from different sources, like numbers, facts, images, or sounds, that can be used to understand something, solve problems, or make decisions. - Data Annotation
The process of labeling data (e.g., tagging images, highlighting text) to create training datasets for artificial intelligence models. - Data Privacy
Protecting personal or sensitive information when using artificial intelligence systems. Teachers must try to ensure student data is handled responsibly and ethically. - Deep Learning
A subset of machine learning where computers use multiple layers of artificial neurons, known as neural networks, to analyze and learn from large amounts of data. Each layer processes the data in increasingly complex ways, allowing the system to recognize patterns, make decisions, and improve over time without explicit programming. This approach is inspired by the way the human brain processes information, enabling tasks such as image and speech recognition, natural language processing, and even playing complex games. - De-identified Data
Data from which personally identifiable information (PII) has been removed or masked, but which could still be linked back to an individual with additional information.
E
- Ethics in AI
The principles guiding the responsible use of artificial intelligence, ensuring fairness, transparency, accountability, and the avoidance of harm. - Explainability
The ability to understand and interpret how an artificial intelligence model makes its decisions, which is important for trust and transparency.
F
- Feedback Loop
The process of using the outcomes of an artificial intelligence model to refine and improve its performance over time.
G
- Generative AI
A type of artificial intelligence that can create new content, such as text, images, music, or even video, by learning from existing data. It uses models like neural networks to generate original material that mimics the patterns and styles found in the data it was trained on.
H
- Human-in-the-Loop
A method where humans work alongside artificial intelligence systems, providing oversight, input, or corrections to improve results.
I
- IoT (Internet of Things)
The network of physical devices connected to the internet, enabling them to collect and share data. artificial intelligence can analyze this data to support learning environments.
M
- Machine Learning
A type of artificial intelligence where computers learn from data to make decisions or predictions without being explicitly programmed. It’s like how teachers help students learn by giving them examples and exercises, allowing them to improve and understand concepts better over time. - Multimodal Prompting
Combining text with images, audio, or video as input.
N
- Natural Language Processing (NLP)
A field of artificial intelligence focused on enabling computers to understand, interpret, and generate human language. Examples include chatbots and translation tools. - Neural Network
A type of machine learning model inspired by the structure of the human brain, consisting of layers of nodes (neurons) that process information to recognize patterns.
O
- One-Shot Prompting
Providing a single example before asking artificial intelligence to generate a response. - Overfitting
A situation where an artificial intelligence model performs well on training data but fails to generalize to new, unseen data. This is particularly important in education because it can lead to tools that are not effective for diverse learners or varying classroom environments.
P
- Personally Identifiable Information (PII)
Information that can directly or indirectly identify a specific individual, such as names, addresses, social security numbers, or student ID numbers. - Prompt
In the context of artificial intelligence, a “prompt” is the text, question, or instructions given to an artificial intelligence system to guide the response or action it provides. By carefully choosing or phrasing a prompt, users can influence the quality and relevance of the AI’s output. - Predictive Modeling
The use of artificial intelligence to analyze data and predict potential future outcomes, such as student performance or trends. - Prompt Engineering
The process of crafting effective questions or instructions to get desired outputs from generative artificial intelligence tools.
R
- Reinforcement Learning
A type of machine learning where an artificial intelligence system learns by trial and error, receiving rewards for correct actions and penalties for incorrect ones.
S
- Supervised Learning
A type of machine learning where the model is trained using labeled data (e.g., photos labeled as “cat” or “dog”) to predict outcomes. For our Teachable Machine tasks, we are labeling photos as “ripe” or “unripe” bananas.
T
- Tokenization
The process of breaking down text into smaller pieces, such as words, sentences, or even individual characters, so a computer can analyze it. In artificial intelligence, tokenization is used to help systems understand and process human language, like in chatbots or language translation tools. - Training Data
The dataset used to teach an artificial intelligence system to perform a specific task. High-quality, diverse training data is essential for creating accurate models. - Transfer Learning
Reusing a pre-trained artificial intelligence model on a new but related task, reducing the amount of training data needed. - Turing Test
A test proposed by Alan Turing to evaluate whether a machine can exhibit intelligent behavior indistinguishable from that of a human.
U
- Unsupervised Learning
A type of machine learning where the model identifies patterns or groupings in data without being given explicit labels.
Z
- Zero-Shot Prompting
Asking the artificial intelligence tool to generate an answer without prior examples or context.