Evaluation & Continuous Improvement
Effective AI integration in K–12 education requires a commitment to ongoing evaluation and iterative refinement. As AI technologies evolve, districts must remain flexible, responsive, and focused on equitable outcomes. Below are key strategies to ensure sustained success:
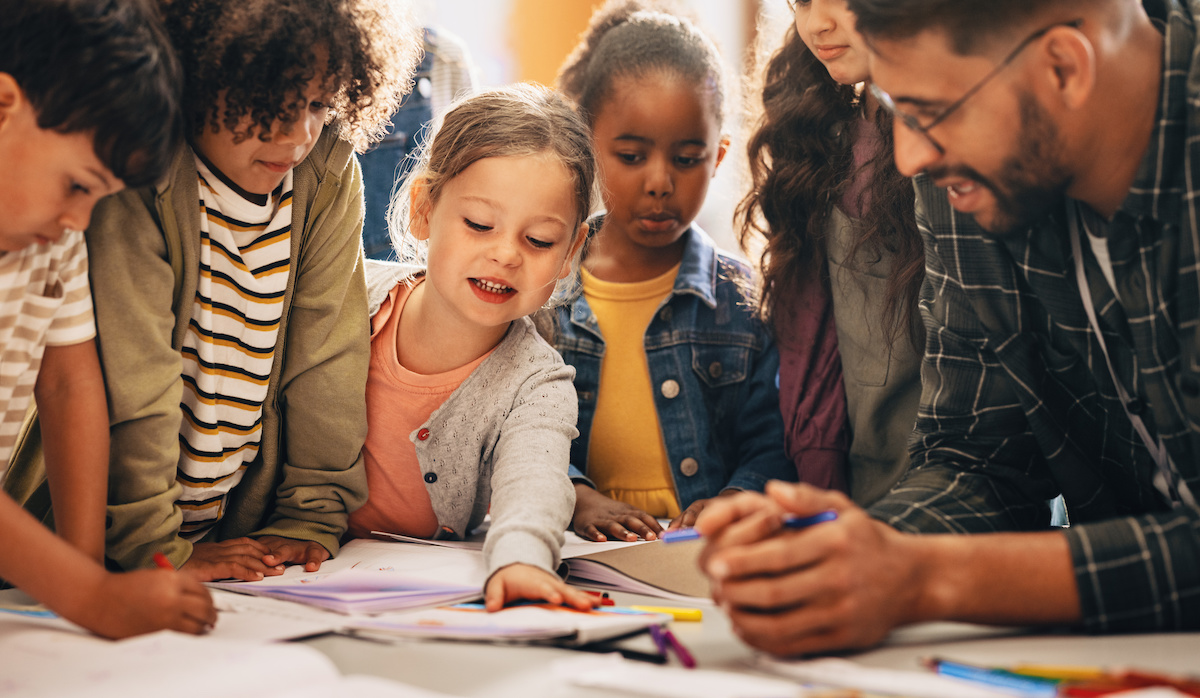
Metrics and Indicators
For any successful AI integration, it is essential to establish clear, measurable outcomes that school and district leaders can track over time. Metrics such as student engagement, teacher adoption rates, and equity indicators (e.g., participation and performance data disaggregated by student demographics) offer a tangible view of AI’s impact on learning. By closely monitoring these figures, administrators can identify whether AI tools are fostering higher student motivation, improving instructional practices, and positively affecting all student groups. Examining trends in these metrics allows districts to fine-tune their support structures—such as professional development, device access, or differentiated instructional strategies—to ensure AI initiatives are meeting set goals and not inadvertently exacerbating existing gaps.
Feedback Loops
Regular feedback from students, teachers, parents, and community stakeholders helps school leaders gauge the real-world effectiveness of AI initiatives and adapt practices accordingly. Periodic surveys, focus groups, and listening sessions provide qualitative insights that enrich quantitative data, revealing successes or areas of concern that raw metrics may miss. Organizing reflective data reviews—where administrators, educators, and IT staff collaboratively examine usage analytics—can also spark meaningful discussion about specific challenges or opportunities. This inclusive approach to feedback promotes a sense of shared ownership over AI integration, ensuring that decisions about resource allocation, training, or policy adjustments remain closely aligned with the lived experiences and needs of the entire school community.
Policy Updates
Because AI tools and regulations evolve rapidly, guidelines surrounding responsible and equitable use must be designed as “living documents.” Districts should conduct scheduled policy audits—perhaps on a semester or annual basis—to review the latest developments in technology, data privacy laws, and community standards. During these reviews, leaders can decide whether to revise acceptable-use policies, data-handling procedures, or academic integrity guidelines in light of newly available AI solutions or emerging concerns. Maintaining open lines of communication with all stakeholders during the update process is vital: prompt, transparent announcements about any policy changes—and the reasoning behind them—foster trust and help educators, students, and families adapt successfully to new norms. By viewing policy as an iterative process, districts remain proactive and prepared, ensuring their AI strategies are both current and responsive to community values.